In the post “What is the nature of time?” the essence of time has been analyzed from the point of view of physics. Several conclusions have been drawn from it, which can be summarized in the following points:
- Time is an observable that emerges at the classical level from quantum reality.
- Time is determined by the sequence of events that determines the dynamics of classical reality.
- Time is not reversible, but is a unidirectional process determined by the sequence of events (arrow of time), in which entropy grows in the direction of the sequence of events.
- Quantum reality has a reversible nature, so the entropy of the system is constant and therefore its description is an invariant.
- The space-time synchronization of events requires an intimate connection of space-time at the level of quantum reality, which is deduced from the theory of relativity and quantum entanglement.
Therefore, a sequence of events can be established which allows describing the dynamics of a classical system (CS) in the following way:
CS = {… Si-2, Si-1, Si, Si+1, Si+2,…}, where Si is the state of the system at instant i.
This perspective has as a consequence that from a perceptual point of view the past can be defined as the sequence {… S-2, S-1}, the future as the sequence {S+1, S+2,…} and the present as the state S0.
At this point it is important to emphasize that these states are perfectly distinguishable from a sequential conception (time) since the amount of information of each state, determined by its entropy, verifies that:
H(Si) < H(Si+1) [1].
Therefore, it seems necessary to analyze how this sequence of states can be interpreted by an observer, the process of perception being a very prominent factor in the development of philosophical theories on the nature of time.
Without going into the foundation of these theories, since we have exhaustive references on the subject [2], we will focus on how the sequence of events produced by the dynamics of a system can be interpreted from the point of view of the mechanisms of perception [3] and from the perspective currently offered by the knowledge on Artificial Intelligence (AI) [4].
Nevertheless, let us make a brief note on what physical time means. According to the theory of relativity, space-time is as if in a vacuum there were a network of clocks and rules of measurement, forming a reference system, in such a way that its geometry depends on the gravitational effects and the relative velocity of the observer’s own reference system. And it is at this point where we can scale in the interpretation of time if we consider the observer as a perceptive entity and establish a relationship between physics and perception.
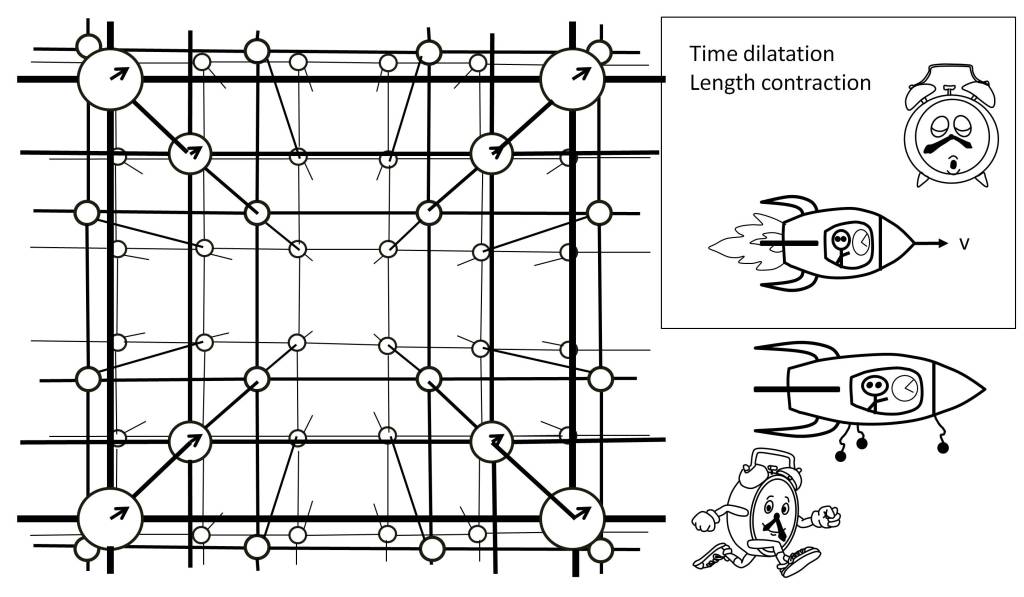
The physical structure of space-time
What we are going to discuss next is whether the sequence of states {… S-2, S-1, S0, S+1, S+2,…} is a physical reality or, on the contrary, is a purely mathematical construction, such that the concept of past, present and future is exclusively a consequence of the perception of this sequence of states. Which means that the only physical reality would be the state of the system S0, and that the sequences {… S-2, S-1} and {S+1, Si+2,…} would be an abstraction or fiction created by the mathematical model.
The contrast between these two views has an immediate consequence. In the first case, in which the sequence of states has physical reality, the physical system would be formed by the set of states {… S-2, S-1, S0, S+1, S+2,…}, which would imply a physical behavior different from the observed universe, which would reinforce the strictly mathematical nature of the sequence of states.
In the second hypothesis there would only be a physical reality determined by the state of the system S0, in such a way that physical time would be an emergent property, consequence of the entropy difference between states that would differentiate them and make them observable.
This conception must be consistent with the theory of relativity, which is possible if we consider that one of the consequences of its postulates is the causality of the system, so that the sequence of events is the same in all reference systems, regardless of the fact that the space-time geometry is different in each of them and therefore the emergent space-time magnitudes are different.
At this point one could posit as fundamental postulates of the theory of relativity the invariance of the sequence of events and covariance. But this is another subject.
Past , present and future
From this physical conception of space-time, the question that arises is how this physical reality determines or conditions an observer’s perception of time.
Thus, in the post “the predictive brain” the ability of neural tissue to process time, which allows higher living beings to interact with the environment, has been indirectly discussed. This requires not only establishing space-time models, but also making space-time predictions [5]. Thus, time perception requires discriminating time intervals of the order of milliseconds to coordinate in real time the stimuli produced by the sensory organs and the actions to activate the motor organs. The performance of these functions is distributed in the brain and involves multiple neural structures, such as the basal ganglia, cerebellum, hippocampus and cerebral cortex [6] [7].
To this we must add that the brain is capable of establishing long-term timelines, as shown by the perception of time in humans [8], in such a way that it allows establishing a narrative of the sequence of events, which is influenced by the subjective interest of those events.
This indicates that when we speak generically of “time” we should establish the context to which we refer. Thus, when we speak of physical time we would be referring to relativistic time, as the time that elapses between two events and that we measure by means of what we define as a clock.
But when we refer to the perception of time, a perceptual entity, human or artificial, interprets the past as something physically real, based on the memory provided by classical reality. But such reality does not exist once the sequence of events has elapsed, since physically only the state S0 exists, so that the states Si, i<0, are only a fiction of the mathematical model. In fact, the very foundation of the mathematical model shows, through chaos theory [9], that it is not possible to reconstruct the states Si, i<0, from S0. In the same way it is not possible to define the future states, although here an additional element appears determined by the increase of the entropy of the system.
With this, we are hypothesizing that the classical universe is S≡S0, and that the states Si, i≠0 have no physical reality (another thing is the quantum universe, which is reversible, so all its states have the same entropy! Although at the moment it is nothing more than a set of mathematical models). Colloquially, this would mean that the classical universe does not have a repository of Si states. In other words, the classical universe would have no memory of itself.
Thus, it is S that supports the memory mechanisms and this is what makes it possible to make a virtual reconstruction of the past, giving support to our memories, as well as to areas of knowledge such as history, archeology or geology. In the same way, state S provides the information to make a virtual construction of what we define as the future, although this issue will be argued later. Without going into details, we know that in previous states we have had some experiences that we store in our memory and in our photo albums.
Therefore, according to this hypothesis it can be concluded that the concepts of past and future do not correspond to a physical reality, since the sequences of states {… S-2, S-1} and {S+1, S+2,…} have no physical reality, since they are only a mathematical artifact. This means that the concepts of past and future are virtual constructs that are materialized on the basis of the present state S, through the mechanisms of perception and memory. The arising question that we will try to answer is the one about how the mechanisms of perception construct these concepts.
Mechanisms of perception
Natural processes are determined by the dynamics of the system in such a way that, according to the proposed model, there is only what we define as present state S. Consequently, if the past and the future have no physical reality, it is worth asking whether plants, inanimate beings are aware of the passage of time.
It is obvious that for humans the answer is yes, otherwise we would not be talking about it. And the reason for this is the information about the past contained in the state S. But this requires the existence of information processing mechanisms that make it possible to virtually construct the past. Similarly, these mechanisms may allow the construction of predictions about future states that constitute the perception of the future [10].
For this, the cognitive function of the brain requires the coordination of neural activity at different levels, from neurons, neural circuits, to large-scale neural networks [7]. As an example of this, the post “The predictive brain” highlights the need to coordinate the stimuli perceived by the sensory organs with the motor organs, in order to be able to interact with the environment. Not only that, but it is essential for the neural tissue to perform predictive processing functions [5], thus overcoming the limitations caused by the response times of neurons.
As already indicated, the perception of time involves several neural structures, which allow the measurement of time at different scales. Thus, the cerebellum allows establishing a time base on the scale of tens of milliseconds [11], analogous to a spatiotemporal metric. Since the dynamics of events is something physical that modifies the state of the system S, the measurement of these changes by the brain requires a physical mechanism that memorizes these changes, analogous to a delay line, which seems to be supported by the cerebellum.
However, this estimation of time cannot be considered at the psychological level as a high-level perceptual functionality, since it is only effective within very short temporal windows, necessary for the performance of functions of an automatic or unconscious nature. For this reason, one could say that time as a physical entity is not perceived by the brain at the conscious level. Thus, what we generally define as time perception is a relationship between events that constitute a story or narrative. This involves processes of attention, memory and consciousness supported in a complex way, involving structures from the basal ganglia to the cerebral cortex, with links between temporal and non-temporal perception mechanisms [12] [13].
Given the complexity of the brain and the mechanisms of perception, attention, memory and self-awareness, it is not possible, at least for the time being, to understand in detail how humans construct temporal stories. Fortunately, we now have AI models that allow to understanding how this can be possible and how stories and narratives can be constructed from the sequential perception of daily life events. A paradigmatic example of this are the “Large Language Models” (LLMs), which based on natural language processing (NLP) techniques and neural networks, are capable of understanding, summarizing, generating and predicting new content and which raise the debate on whether human cognitive capabilities could emerge in these generic models, if provided with sufficient processing resources and training data [14].
Without delving into this debate, today anyone can verify through this type of applications (ChatGPT, BARD, Claude, etc.) how a completely consistent story can be constructed, both in its content and in its temporal plot, from the human experiences reflected in written texts with which these models have been trained.
Taking these models as a reference provides solid evidence on perception in general and on the perception of time in particular. However, it should be noted that these models also show how new properties emerge in their behavior as their complexity grows [15]. This gives a clue as to how new perceptual capabilities or even concepts such as self-awareness may emerge, although this last term is purely speculative, and that in the event that this ends up being the case, it raises the problem discussed in the post “Consciousness from the AI point of view” concerning how to know that an entity is self-aware.
But returning to the subject at hand, what is really important from the point of view of the perception of the passage of time is how the timeline of stories or narratives is a virtual construction that transcends physical time. Thus, the chronological line of events does not refer to a measure of physical time, but is a structure in which a hierarchy or order is established in the course of events.
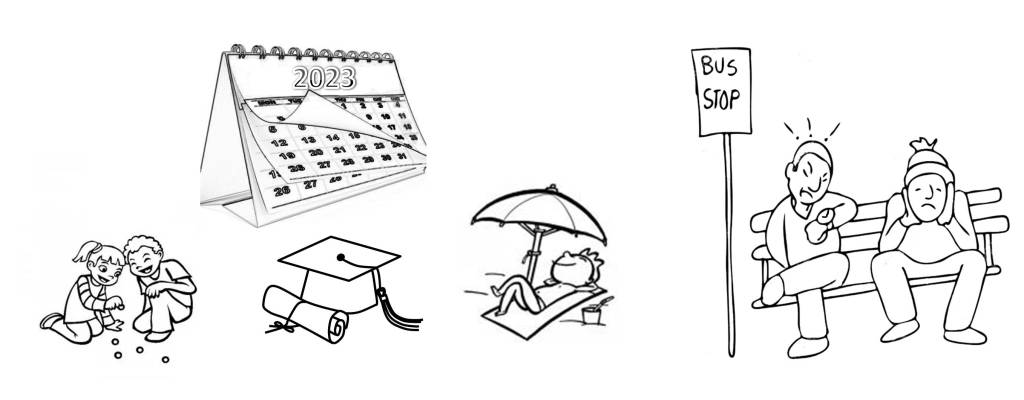
Virtual perception of time
It can therefore be concluded that the brain only needs to measure physical time in the very short term, in order to be able to interact with the physical environment. But from this point on, all that is needed is to establish a chronological order without a precise reference to physical time. Thus we can refer to an hour, day, month, year, or a reference to another event as a way of ordering events, but always within a purely virtual context. This is one of the reasons for how the passage of time is perceived, so that virtual time will be extended according to the amount of information or relevance of events, something that is evident in playful or stressful situations [16].
Conclusions
The first conclusion that results from the above analysis is the existence of two conceptions of time. One is the one related to physical time that corresponds to the sequence of states of a physical system and the other is the one corresponding to the stimuli produced by this sequence of states on a perceptual intelligence.
Both concepts are elusive when it comes to understanding them. We are able to measure physical time with great precision. However, the theory of relativity shows space-time as an emergent reality that depends on the reference system. On the other hand, the synchronization of clocks and the establishment of a space-measuring structure may seem somewhat contrived, oriented simply to the understanding of space-time from the point of view of physics. On the other hand, the compression of cognitive processes still has many unknowns, although new developments in AI allow us to intuit its foundation, which sheds some light on the concept of psychological time.
The interpretation of time as the sequence of events or states occurring within a reference system is consistent with the theory of relativity and also allows for a simple justification of the psychological perception of time as a narrative.
The hypothesis that the past and the future have no physical reality and that, therefore, the universe keeps no record of the sequence of states, supports the idea that these concepts are an emergent reality at the cognitive level, so that the conception of time at the perceptual level would be based on the information contained in the current state of the system, exclusively.
From the point of view of physics this hypothesis does not contradict any physical law. Moreover, it can be considered fundamental in the theory of relativity, since it assures a causal behavior that would solve the question of temporal irreversibility and the impossibility of traveling both to the past and to the future. Moreover, invariance in the time sequence supports the concept of causality, which is fundamental for the emergent system to be logically consistent.
References
[1] | F. Schwabl, Statistical Mechanics, pp. 491-494, Springer, 2006. |
[2] | N. Emery, N. Markosian y M. Sullivan, «”Time”, The Stanford Encyclopedia of Philosophy (Winter 2020 Edition), Edward N. Zalta (ed.), URL = <https://plato.stanford.edu/archives/win2020/entries/time/>,» [En línea]. |
[3] | E. R. Kandel, J. H. Schwartz, S. A. Siegenbaum y A. J. Hudspeth, Principles of Neural Science, The McGraw-Hill, 2013. |
[4] | F. Emmert-Streib, Z. Yang, S. Tripathi y M. Dehmer, «An Introductory Review of Deep Learning for Prediction Models With Big Data,» Front. Artif. Intell., 2020. |
[5] | W. Wiese y T. Metzinger, «Vanilla PP for philosophers: a primer on predictive processing.,» In Philosophy and Predictive Processing. T. Metzinger &W.Wiese, Eds., pp. 1-18, 2017. |
[6] | J. Hawkins y S. Ahmad, «Why Neurons Have Tousands of Synapses, Theory of Sequence Memory in Neocortex,» Frontiers in Neural Circuits, vol. 10, nº 23, 2016. |
[7] | S. Rao, A. Mayer y D. Harrington, «The evolution of brain activation during temporal processing.,» Nature Neuroscience, vol. 4, p. 317–323, 2001. |
[8] | V. Evans, Language and Time: A Cognitive Linguistics Approach, Cambridge University Press, 2013. |
[9] | R. Bishop, «Chaos: The Stanford Encyclopedia of Philosophy, Edward N. Zalta (ed).,» Bishop, Robert, “Chaos”, The Stanford Encyclopedia of Philosophy (Spring 2017 Edition), Edward N. Zalta (ed.), 2017. [En línea]. Available: https://plato.stanford.edu/archives/spr2017/entries/chaos/. [Último acceso: 7 9 2023]. |
[10] | A. Nayebi, R. Rajalingham, M. Jazayeri y G. R. Yang, «Neural Foundations of Mental Simulation: Future Prediction of Latent Representations on Dynamic Scenes,» arXiv.2305.11772v2.pdf, 2023. |
[11] | R. B. Ivry, R. M. Spencer, H. N. Zelaznik y J. Diedrichsen, «Ivry, Richard B., REBECCA M. Spencer, Howard N. Zelaznik and Jörn Diedrichsen. The Cerebellum and Event Timing,» Ivry, Richard B., REBECCA M. Spencer, Howard N. Zelaznik and Jörn DiedrichAnnals of the New York Academy of Sciences, vol. 978, 2002. |
[12] | W. J. Matthews y W. H. Meck, «Temporal cognition: Connecting subjective time to perception, attention, and memory.,» Psychol Bull., vol. 142, nº 8, pp. 865-907, 2016. |
[13] | A. Kok, Functions of the Brain: A Conceptual Approach to Cognitive Neuroscience, Routledge, 2020. |
[14] | J. Wei, Y. Tay, R. Bommasani, C. Raffel, B. Zoph, S. Borgeaud, D. Yogatama, M. Bosma, D. Zhou, D. Metzler, E. H. Chi, T. Hashimoto, O. Vinyals, P. Liang, J. Dean y W. Fedus, «Emergent Abilities of Large Language Models,» Transactions on Machine Learning Research. https://openreview.net/forum?id=yzkSU5zdwD, 2022. |
[15] | T. Webb, K. J. Holyoak y H. Lu, «Emergent Analogical Reasoning in Large Language Models,» Nature Human Behaviour, vol. 7, p. 1526–1541, 3 8 2023. |
[16] | P. U. Tse, J. Intriligator, J. Rivest y P. Cavanagh, «Attention and the subjective expansion of time,» Perception & Psychophysics, vol. 66, pp. 1171-1189, 2004. |