While significant progress has been made in the field of neuroscience and in particular in the neural circuits that support perception and motor activity, the understanding of neural structures, how they encode information, and establish the mechanisms of learning is still under investigation.
Digital audio and image processing techniques and advances in artificial intelligence (AI) are a source of inspiration for understanding these mechanisms. However, it seems clear that these ideas are not directly applicable to brain functionality.
Thus, for example, the processing of an image is static, since digital sensors provide complete images of the scene. In contrast, the information encoded by the retina is not homogeneous, with large differences in resolution between the fovea and the surrounding areas, so that the image composition is necessarily spatially segmented.
But these differences are much more pronounced if we consider that this information is dynamic in time. In the case of digital video processing, it is possible to establish a correlation of the images that make up a sequence. A correlation that in the case of the visual system is much more complex, due to the spatial segmentation of the images and how this information is obtained using the saccadic movements of the eyes.
The information generated by the retina is processed by the primary visual cortex (V1) which has a well-defined map of spatial information and also performs simple feature recognition functions. This information progresses to the secondary visual cortex (V2) which is responsible for composing the spatial information generated by saccadic eye movement.
This structure has been the dominant theoretical framework, in what has been termed the hierarchical feedforward model [1]. However, certain neurons in V1 and V2 regions have been found to have a surprising response. They seem to know what is going to happen in the immediate future, activating as if they could perceive new visual information without it having been produced by the retina [2], in what is defined as Predictive Processing (PP) [3], and which is gaining influence in cognitive neuroscience, although it is criticized for lacking empirical support to justify it.
For this reason, the aim of this post is to analyze this behavior from the point of view of signal processing techniques and control systems, which show that the nervous system would not be able to interact with the surrounding reality unless it performs PP functions.
A brief review of control systems
The design of a control system is based on a mature technique [4], although the advances in digital signal processing produced in the last decades allow the implementation of highly sophisticated systems. We will not go into details about these techniques and will only focus on the aspects necessary to justify the possible PP performed by the brain.
Thus, a closed-loop control system is composed of three fundamental blocks:
- Feedback: This block determines the state of the target under control.
- Control: Determines the actions to be taken based on the reference and the information on the state of the target.
- Process: Translates the actions determined by the control to the physical world of the target.
The functionality of a control system is shown in the example shown in the figure. In this case the reference is the position of the ball and the target is for the robot to hit the ball accurately.
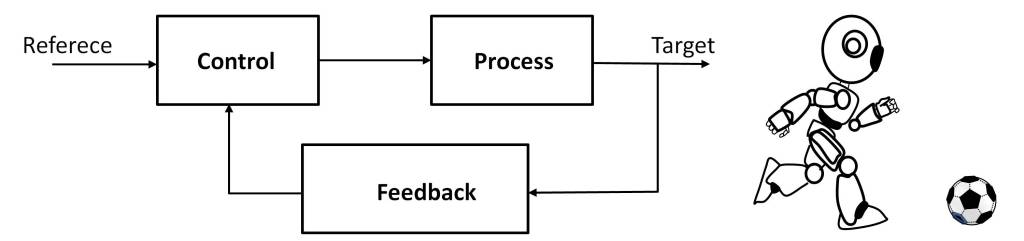
The robot sensors must determine in real-time the relative position of the ball and all the parameters that define the robot structure (feedback). From these, the control must determine the robot motion parameters necessary to reach the target, generating the control commands that activate the robot’s servomechanisms.
The theoretical analysis of this functional structure allows determining the stability of the system, which establishes its capacity to correctly develop the functionality for which it has been designed. This analysis shows that the system can exhibit two extreme cases of behavior. To simplify the reasoning, we will eliminate the ball and assume that the objective is to reach a certain position.
In the first case, we will assume that the robot has a motion capability such that it can perform fast movements without limitation, but that the measurement mechanisms that determine the robot’s position require a certain processing time Δt. As a consequence, the decisions of the control block are not in real-time since the decisions at t = ti actually correspond to t = ti-Δt, where Δt is the time required to process the information coming from the sensing mechanisms. Therefore, when the robot approaches the reference point the control will make decisions as if it were somewhat distant, which will cause the robot to overshoot the position of the target. When this happens, the control should correct the motion by turning back the robot’s trajectory. This behavior is defined as an underdamped regime.
Conversely, if we assume that the measurement system has a fast response time, such that Δt≊0, but that the robot’s motion capability is limited, then the control will make decisions in real-time, but the approach to the target will be slow until the target is accurately reached. Such behavior is defined as an overdamped regime.
At the boundary of these two behaviors is the critically damped regime that optimizes the speed and accuracy to reach the target. The behavior of these regimes is shown in the figure.

Formally, the above analysis corresponds to systems in which the functional blocks are linear. The development of digital processing techniques allows the implementation of functional blocks with a nonlinear response, resulting in much more efficient control systems in terms of response speed and accuracy. In addition, they allow the implementation of predictive processing techniques using the laws of mechanics. Thus, if the reference is a passive entity, its trajectory is known from the initial conditions. If it is an active entity, i.e. it has internal mechanisms that can modify its dynamics, heuristic functions, and AI can be used [5].
The brain as a control system
As the figure below shows, the ensemble formed by the brain, the motor organs, and the sensory organs comprises a control system. Consequently, this system can be analyzed with the techniques of feedback control systems.

For this purpose, it is necessary to analyze the response times of each of the functional blocks. In this regard, it should be noted that the nervous system has a relatively slow temporal behavior [6]. Thus, for example, the response time to initiate movement in a 100-meter sprint is 120-165 ms. This time is distributed in recognizing the start signal, the processing time of the brain to interpret this signal and generate the control commands to the motor organs, and the start-up of these organs. In the case of eye movements toward a new target, the response time is 50-200 ms. These times give an idea of the processing speed of the different organs involved in the different scenarios of interaction with reality.
Now, let’s assume several scenarios of interaction with the environment:
- A soccer player intending to hit a ball moving at a speed of 10 km/hour. In a time of 0.1 s. the ball will have moved 30 cm.
- A tennis player who must hit a ball moving at 50 km/hour. In a time of 0.1 s. the ball will have displaced 150 cm.
- Grip a motionless cup by moving the hand at a speed of 0.5 m/s. In a time of 0.1 s. the hand will have moved 5 cm.
These examples show that if the brain is considered as a classical control system, it is practically impossible to obtain the necessary precision to justify the behavior of the system. Thus, in the case of the soccer player, the information obtained by the brain from the sensory organs, in this case, the sight, will be delayed in time, providing a relative position of the foot concerning the ball with an error of the order of centimeters, so that the ball strike will be very inaccurate.
The same reasoning can be made in the case of the other two proposed scenarios, so it is necessary to investigate the mechanisms used by the brain to obtain an accuracy that justifies its actual behavior, much more accurate than that provided by a control system based on the temporal response of neurons and nerve tissue.
To this end, let’s assume the case of grasping the cup, and let’s do a simple exercise of introspection. If we close our eyes for a moment we can observe that we have a precise knowledge of the environment. This knowledge is updated as we interact with the environment and the hand approaches the cup. This spatiotemporal behavior allows predicting with the necessary precision what will be the position of the hand and the cup at any moment, despite the delay produced by the nervous system.
To this must be added the knowledge acquired by the brain about space-time reality and the laws of mechanics. In this way, the brain can predict the most probable trajectory of the ball in the tennis player’s scenario. This is evident in the importance of training in sports activities since this knowledge must be refreshed frequently to provide the necessary accuracy. Without the above prediction mechanisms, the tennis player would not be able to hit the ball.
Consequently, from the analysis of the behavior of the system formed by the sensory organs, the brain, and the motor organs, it follows that the brain must perform PP functions. Otherwise, and as a consequence of the response time of the nervous tissue, the system would not be able to interact with the environment with the precision and speed shown in practice. In fact, to compensate for the delay introduced by the sensory organs and their subsequent interpretation by the brain, the brain must predict and advance the commands to the motor organs in a time interval that can be estimated at several tens of milliseconds.
The neurological foundations of prediction
As justified in the previous section, from the temporal response of the nervous tissue and the behavior of the system formed by the sensory organs, the brain, and the motor organs, it follows that the brain must support two fundamental functions: encoding and processing reference frames of the surrounding reality and performing Predictive Processing.
But what evidence is for this behavior? It has been known for several decades that there are neurons in the entorhinal cortex and hippocampus that respond to a spatial model, called grid cells [7]. But recently it has been shown that in the neocortex there are structures capable of representing reference frames and that these structures can render both a spatial map and any other functional structure needed to represent concepts, language, and structured reasoning [8].
Therefore, the question to be resolved is how the nervous system performs PP. As already advanced, PP is a disputed functionality because of its lack of evidence. The problem it poses is that the number of neurons that exhibit predictive behavior is very small compared to the number of neurons that are activated as a consequence of a stimulus.
The answer to this problem may lie in the model proposed by Jeff Hawkins and Subutai Ahmad [9] based on the functionality of pyramidal neurons [10], whose function is related to motor control and cognition, areas in which PP should be fundamental.
The figure below shows the structure of a pyramidal neuron, which is the most common type of neuron in the neocortex. The dendrites close to the cell body are called proximal synapses so that the neuron is activated if they receive sufficient excitation. The nerve impulse generated by the activation of the neuron propagates to other neurons through the axon, which is represented by an arrow.
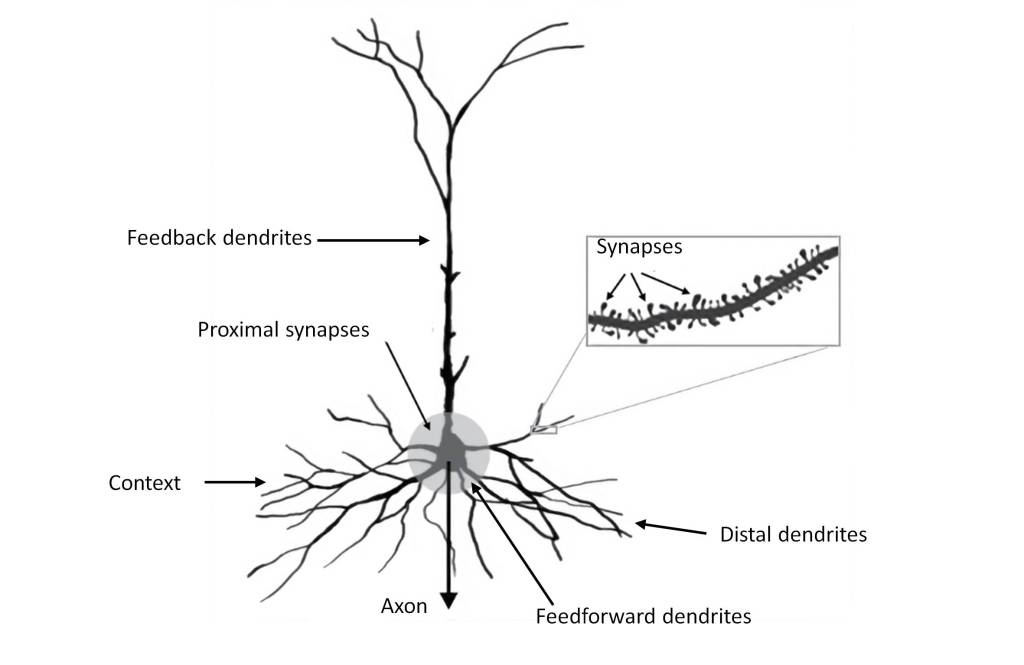
This description corresponds to a classical view of the neuron, but pyramidal neurons have a much more complex structure. The dendrites radiating from the central zone are endowed with hundreds or thousands of synapses, called distal synapses so approximately 90% of the synapses are located on these dendrites. Also, the upper part of the figure shows dendrites that have a longer reach, which have feedback functionality.
The remarkable thing about this type of neuron is that if a group of synapses of a distal dendrite close to each other receives a signal at the same time, a new type of nerve impulse is produced that propagates along the dendrite until it reaches the body of the cell. This causes an increase in the voltage of the cell, but without producing its activation, so it does not generate a nerve impulse towards the axon. The neuron remains in this state for a short period, returning to its relaxed state.
The question is: What is the purpose of these nerve impulses from the dendrites if they are not powerful enough to produce cell activation? This has been an unknown that is intended to be solved by the model proposed by Hawkins and Ahmad [9], which proposes that the nerve impulses in the distal dendrites are predictions.
This means that a dendritic impulse is produced when a set of synapses close to each other on a distal dendrite receive inputs at the same time, and it means that the neuron has recognized a pattern of activity determined by a set of neurons. When the pattern of activity is detected, a dendritic impulse is created, which raises the voltage in the cell body, putting the cell into what we call a predictive state.
The neuron is then ready to fire. If a neuron in the predictive state subsequently receives sufficient proximal input to create an action potential to fire it, then the neuron fires slightly earlier than it would if the neuron were not in the predictive state.
Thus, the prediction mechanism is based on the idea that multiple neurons in a minicolumn [11] participate in the prediction of a pattern, all of them entering a prediction state, such that when one of them fires it inhibits the firing of the rest. This means that in a minicolumn hundreds or thousands of predictions are made simultaneously over a certain control scenario, such that one of the predictions will prevail over the rest, optimizing the accuracy of the process. This justifies the fact of the small number of predictive events observed versus the overall neuronal activity and also explains why unexpected events or patterns produce greater activity than more predictable or expected events.
If the neural structure of the minicolumns is taken into account, it is easy to understand how this mechanism involves a large number of predictions for the processing of a single pattern, and it can be said that the brain is continuously making predictions about the environment, which allows real-time interaction.
The PP from the point of view of AI
According to the above analysis, it can be concluded that the PP performed by the brain within a time window, of the order of tens of milliseconds, is fundamental for the interaction with the surrounding reality, synchronizing this reality with the perceived reality. But this ability to anticipate perceived events requires other mechanisms such as the need to establish reference frames as well as the ability to recognize patterns.
In the subject raised, it is evident the need to have reference frames in which objects can be represented, such as the dynamic position of the motor organs and of the objects with which to interact. In addition to this, the brain must be able to recognize such objects.
But these capabilities are common to all types of scenarios, although it is perhaps more appropriate to use the term model as an alternative to a reference frame since it is a more general concept. Thus, for example, in verbal communication, it is necessary to have a model that represents the structure of language, as well as an ability to recognize the patterns encoded in the stimuli perceived through the auditory system. In this case, the PP must play a fundamental role, since prediction allows for greater fluency in verbal communication, as is evident when there are delays in a communication channel. This is perhaps most evident in the synchronism necessary in musical coordination.
The enormous complexity of the nervous tissue and the difficulty to empirically identify these mechanisms can be an obstacle to understanding their behavior. For this reason, AI is a source of inspiration [12] since, using different neural network architectures, it shows how models of reality can be established and predictions can be made about this reality.
It should be noted that these models do not claim to provide realistic biological models. Nevertheless, they are fundamental mathematical models in the paradigm of machine learning and artificial intelligence and are a fundamental tool in neurological research. In this sense, it is important to highlight that PP is not only a necessary functionality for the temporal prediction of events, but as shown by artificial neural networks pattern recognition is intrinsically a predictive function.
This may go unnoticed in the case of the brain since pattern recognition achieves such accuracy that it makes the concept of prediction very diluted and appears to be free of probabilistic factors. In contrast, in the case of AI, mathematical models make it clear that pattern recognition is probabilistic in nature and practical results show a diversity of outcomes.
This diversity depends on several factors. Perhaps the most important is its state of development, which can still be considered very primitive, compared to the structural complexity, processing capacity, and energy efficiency of the brain. This means that AI applications are oriented to specific cases where it has shown its effectiveness, such as in health sciences [13] or in the determination of protein structures [14].
But without going into a deeper analysis of these factors, what can be concluded is that the functionality of the brain is based on the establishment of models of reality and the prediction of patterns, one of its functions being temporal prediction, which is the foundation of PP.
References
[1] | J. DiCarlo, D. Zoccolan y N. Rust, «How does the brain solve visual object recognition?,» Neuron, vol. 73, pp. 415-434, 2012. |
[2] | A. Clark, «Whatever next? Predictive brains, situated agents, and the future of cognitive science,» Behav. Brain Sci., vol. 34, p. 181–204, 2013. |
[3] | W. Wiese y T. Metzinger, «Vanilla PP for philosophers: a primer on predictive processing.,» In Philosophy and Predictive Processing. T. Metzinger &W.Wiese, Eds., pp. 1-18, 2017. |
[4] | G. F. Franklin, J. D. Powell y A. Emami-Naeini, Feedback Control of Dynamic Systems, Pearson; 8a edición, 2019. |
[5] | C. Su, S. Rakheja y H. Liu, «Intelligent Robotics and Applications,» de 5th International Conference, ICIRA, Proceedings, Part II, Montreal, QC, Canada, 2012. |
[6] | A. Roberts, R. Borisyuk, E. Buhl, A. Ferrario, S. Koutsikou, W.-C. Li y S. Soffe, «The decision to move: response times, neuronal circuits and sensory memory in a simple vertebrate,» Proc. R. Soc. B, vol. 286: 20190297, 2019. |
[7] | M. B. Moser, «Grid Cells, Place Cells and Memory,» de Nobel Lecture. Aula Medica, Karolinska Institutet, Stockholm, http://www.nobelprize.org/prizes/medicine/2014/may-britt-moser/lecture/, 2014. |
[8] | M. Lewis, S. Purdy, S. Ahmad y J. Hawkings, «Locations in the Neocortex: A Theory of Sensorimotor Object Recognition Using Cortical Grid Cells,» Frontiers in Neural Circuits, vol. 13, nº 22, 2019. |
[9] | J. Hawkins y S. Ahmad, «Why Neurons Have Tousands of Synapses, Theory of Sequence Memory in Neocortex,» Frontiers in Neural Circuits, vol. 10, nº 23, 2016. |
[10] | G. N. Elston, «Cortex, Cognition and the Cell: New Insights into the Pyramidal Neuron and Prefrontal Function,» Cerebral Cortex, vol. 13, nº 11, p. 1124–1138, 2003. |
[11] | V. B. Mountcastle, «The columnar organization of the neocortex,» Brain, vol. 120, p. 701–722, 1997. |
[12] | F. Emmert-Streib, Z. Yang, S. Tripathi y M. Dehmer, «An Introductory Review of Deep Learning for Prediction Models With Big Data,» Front. Artif. Intell., 2020. |
[13] | A. Bohr y K. Memarzadeh, Artificial Intelligence in Healthcare, Academic Press, 2020. |
[14] | E. Callaway, «‘It will change everything’: DeepMind’s AI makes gigantic leap in solving protein structures,» Nature, nº 588, pp. 203-204, 2020. |